Keywords:
By David Wold
Understanding the spatial context of the changes that occur in molecular and cellular interactions during disease is critical for the development of effective diagnostics and novel therapies. Spatial biology, or spatial omics, is the recently coined blanket term covering the science and technologies that allow researchers and clinicians to study these changes: to visualize and study the interactions of individual molecules, tissues, and cells in context, in three dimensions, over time.1-3 The question is: with such a huge variety of technologies and applications available, which spatial biology applications are relevant to your projects, and could be implemented in your lab?
Overview of spatial biology technologies and workflows in the diagnosis, monitoring and treatment of disease, from sample prep to analysis.
Spatial biology: why is it so popular?
Spatial “omics” is now mentioned in hundreds of publications describing methods to obtain and analyze spatially resolved information for any omics data type, from single molecules to single cells, tissues, and even whole organs and organisms.1-3 Spatial methods are being developed at breakneck speed, with the most prominent example being spatial transcriptomics, which has seen an explosion of publications since being recognized as the “method of the year 2020” in Nature Methods.1,4-7 Progress has also accelerated in several other spatial omics modalities, including genomics, epigenomics, proteomics, and metabolomics.
As its name implies, spatial omics is multidisciplinary, with methods based on both in situ and ex situ approaches. The in situ methods rely on imaging, be that highly multiplex fluorescence microscopy or imaging mass spectrometry, whereas the ex situ methods are based on either next-generation sequencing (NGS) or mass spectrometry. Figure 1 summarizes how these various technologies are converging and becoming more integrated.
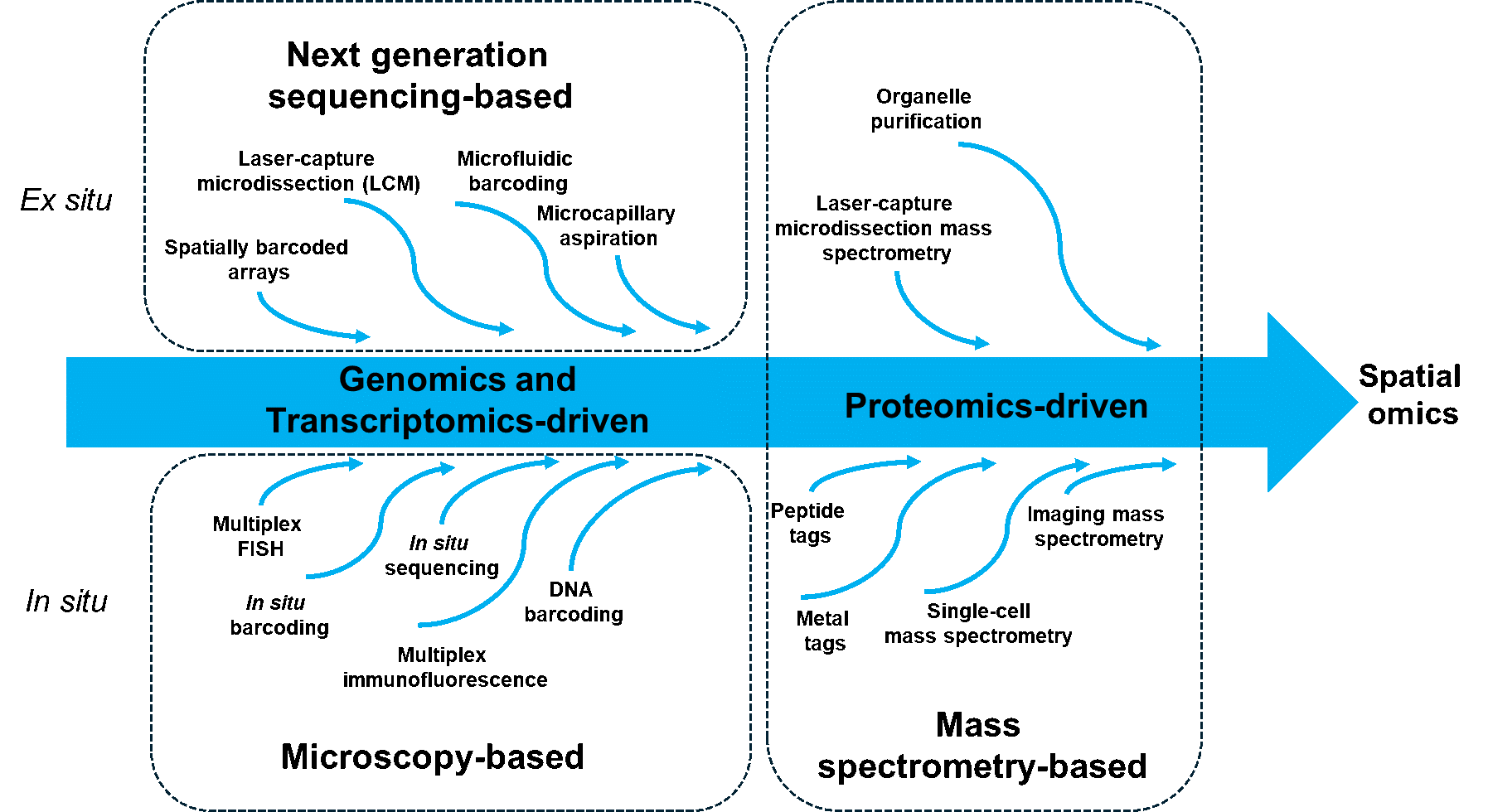
Figure 1. Unraveling the core capabilities of spatial omics technologies, stemming from traditional omics, microscopy, and mass spectrometry. Initially focused on only one component, many are increasingly combined into multi-omics domains (adapted from Alexandrov et al., 2023).
The recent developments in spatial biology represent a massive paradigm shift, enabling molecular profiles to be mapped within the structural and anatomical context of tissues and organisms. We can only touch on them here: there are several excellent reviews on spatial omics, a few of which you will find in the bibliography.8-12 Some key opportunities that can be unlocked with spatial omics include:
- Understanding how gene regulation is modulated by cells' specific spatial microenvironments e.g. looking at tumor heterogeneity
- Discovering and developing novel biomarkers and therapeutic targets for cancer, neurodegenerative and other diseases
- Characterizing drug distribution/resistance based on spatial molecular signatures
- Creating integrated atlases to comprehensively map gene, RNA and protein expression in individual cells and cell types across whole organisms
Spatial omics: the prequel
The most basic form of spatial biology has been around for decades, with the advent of immunohistochemistry (IHC) in the 40s, and in situ hybridization (ISH) in the 70s.1 This is when dyes — and later, fluorescent probes — were first applied to thin layers of tissue on microscope slides to detect proteins and nucleic acids. IHC uses antibodies to detect the location of proteins and other antigens in tissue sections, and ISH is used to look for specific sequences of DNA or RNA. Fluorescence microscopy is used for spatial mapping of specific genes, mapping gene expression (e.g. RNA-FISH), or localization of proteins (e.g. immunofluorescence) and metabolites (e.g. using fluorescent metabolite sensors).1
The move towards an “omics” view of this same sample would mean scaling up the capabilities of these techniques by several orders of magnitude, to enable the detection and quantification of 100s or 1000s of different genes, RNAs, and proteins, whilst retaining their spatial information. Now, whilst ISH and IHC can be multiplexed according to the number of dyes that can be spectrally differentiated during a single image “take”, a sequential approach of some kind is required if we want to look at more than a few genes or proteins at any one time. This limitation has led to the development of a whole slew of in situ labeling methods to increase multiplexing.2
Some examples are:
- non-microscopic methods such as imaging mass spectrometry (IMS) to detect analytes
- cyclic imaging methods, with cycles of staining and dye removal to achieve the required complexity, using labelled antibodies or oligonucleotides
- harnessing the power of high-throughput DNA sequencing and barcoding.
Another part of this evolutionary journey began with single-cell analysis, where “omics” technologies are applied to disaggregated tissues, such as in single-cell sequencing, single-cell mass spectrometry, or single-cell imaging. However, since biological processes happen in a spatial context, methods were sought to use these technologies to go beyond single cells and look at their function in their three-dimensional (3D) context, both in situ and ex situ, to arrive at where we are today.
Put simply, by giving single cells or groups of cells some kind of location label, such as a unique positional barcode, and then applying single-cell analysis techniques, the data arising from these experiments can be re-assembled to give spatial insights into gene and protein expression on the level of the tissue or organism, rather than on the level of single isolated cells.
Spatial transcriptomics and genomics
The ultimate goal of “spatial transcriptomics” is to measure the abundance of every gene and gene isoform, at subcellular resolution, in a whole tissue sample in three dimensions.2 However, technologies currently focus on histological sections of various thicknesses, so they are typically 2D rather than 3D methods. Let us look at four of the more common types of spatial genomics and transcriptomic methods.
(i) Microdissection
In microdissection, specific areas of tissue are physically isolated or otherwise marked so that their DNA or RNA can be extracted and processed. Laser-capture microdissection (LCM) is routinely used to isolate small areas (tens to hundreds of cells) for RNA-sequencing (RNA-seq) and can reach single-cell resolution.13,14 This technique offers deep profiling but has comparatively low throughput (<100s of locations) because each selected area has to be collected and processed individually, although this can be partly overcome with advances in barcoding.2,13
(ii) Combinatorial FISH
Combinatorial fluorescencein situ hybridization (FISH) can be combined with single-molecule imaging to allow the quantification of the number of mRNA molecules present in a given area of a tissue.2,15,16 This combination of techniques is ultra-sensitive, being able to detect single molecules per cell, and can theoretically be used to profile millions of targets, as opposed to tens or hundreds. Molecules are detected through hybridization and identified according to patterns of fluorescence signal. Multiplexed error robust FISH (MERFISH), sequential FISH (seqFISH), and seqFISH+ use combinatorial labeling schemes to assign each transcript to a barcode, consisting of a subset of hybridization probes that are detected in cycles of imaging and release. 2,15,16 We will discuss some of these methods and workflows in our next article.
(iii) in situ sequencing of mRNA molecules
in situ sequencing technologies execute sequencing reactions directly on DNA amplicon clusters (“rolonies”) derived from mRNAs within tissue sections, allowing spatial mapping of transcripts in their native context.2,17,18 Common steps include rolling circle amplification (RCA) to generate rolonies, with one per mRNA molecule for quantification, and sequencing by ligation rather than synthesis, limiting reads to ~30 nucleotides. Specific methods include in situ sequencing (ISS) and fluorescencein situ sequencing (FISSEQ). While enabling spatially-resolved transcriptomics, in situ methods still face limitations in sensitivity, throughput, and read lengths when compared to cyclic fluorescence techniques like MERFISH.
(iv) spatial barcoding methods
In these methods, the DNA and mRNAs in a given area are linked to a specific predetermined DNA “barcode”, which allows them to be computationally mapped back to a spatial location after bulk sequencing.19,20 Spots of barcoded oligo captors are printed on a microarray, with each spot containing a unique spatial barcode along with a poly(T) sequence to capture polyadenylated mRNAs. A tissue section is then placed on the array, allowing mRNAs to diffuse to the barcoded oligos and be reverse transcribed in situ to yield spatially barcoded cDNAs. These are then sequenced using standard RNA-seq. Key advantages include long reads, lack of reliance on complex imaging, high speed, and parallelization. However, limitations include low spatial resolution, high costs per sample, and low RNA capture efficiency compared to other spatial methods.
A word about spatial proteomics
The primary spatial proteomics approaches are mass cytometry and cyclic fluorescence imaging methods.1,2,21,22 Mass cytometry techniques like imaging mass cytometry (IMC) and multiplexed ion beam imaging (MIBI) use antibodies conjugated to lanthanide metals. During imaging, a laser ablates the sample, and a mass spectrometer detects the released metals to map their distributions, allowing multiplexed quantification of over 40 proteins.
An alternative involves cyclic fluorescence imaging over repeated rounds. Some methods, such as CODEX (codetection by imaging), bind all fluorescently labeled antibodies together initially. While slower per sample than mass cytometry, fluorescence techniques have higher data-generating capacity by parallelizing sample staining. Mass cytometry is somewhat limited by the scarcity of pure metals. Both mass cytometry and cyclic fluorescence imaging have enabled highly multiplexed spatial proteomics studies, particularly for characterizing tumor microenvironments in immunology and cancer, even if they are limited in terms of the number of proteins that can be analyzed.
From small samples to big data
Data acquisition is often considered to be the most challenging task in spatial omics. However, the manipulation, analysis, and visualization of data are equally important, if not more so.1,2,11,23 Spatial profiling methods generate enormous datasets (often many terabytes), which are influenced and biased by various factors, and an experiment that costs thousands of dollars can easily become entirely useless without the appropriate analytical tools. Figure 2 illustrates the complexity of various spatial omics workflows and hence the concurrent and downstream analysis.
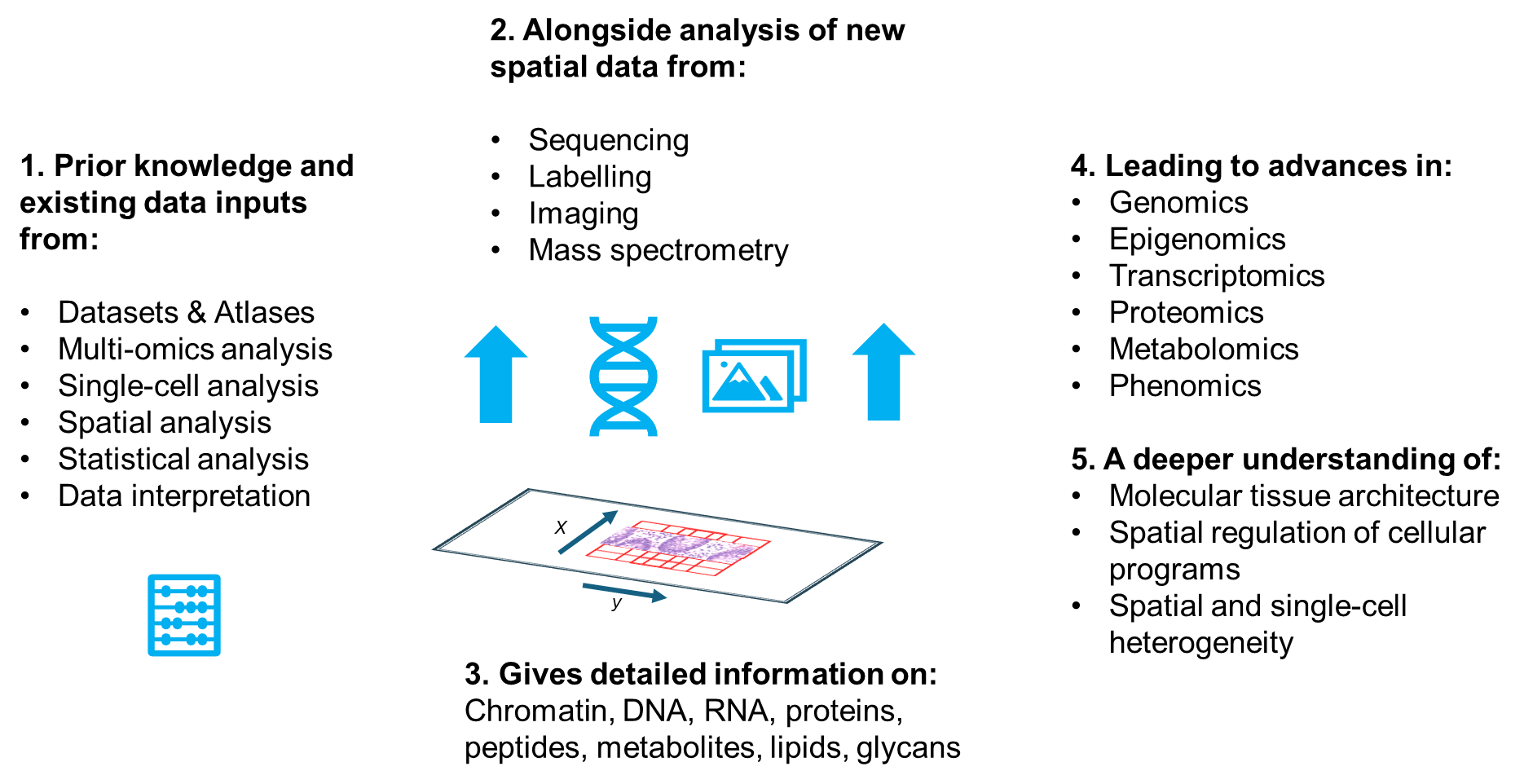
Figure 2. The data analysis associated with spatial omics workflows is extremely complex. It necessitates the spatial identification of gene and protein expression within the sample, followed by filtering, normalization, clustering and identification of differentially expressed genes and proteins.
The data analysis workflow will depend on whether the core acquisition modality is imaging or next-generation sequencing.2 For imaging, the images taken at different cycles and across multiple fields of view require preprocessing through stitching, background correction, and realignment with high accuracy (image registration). To achieve image registration, easily identifiable "fiducial marks" are used. These marks can be either point signals produced by the protocol itself (ExSeq and STARmap), embedded fluorescent microbeads (MERFISH and seqFISH), or the pattern of cell nuclei after intercalator staining (multiplexed IHC).1,2
In single-molecule imaging, the spots with signals need to be identified and decoded to assign them to a biomolecule identification, usually a transcript. After this, images are segmented to define areas corresponding to each cell. The number of spots or signal intensity is then integrated over the cell masks, generating an area-by-features matrix. In contrast, spatial barcoding methods produce datasets in the form of sequencing output. These datasets are preprocessed and parsed to assign each read to two things: a coordinate in space through the location barcodes and a biomolecule's ID by mapping to a reference, such as a transcriptome.2
Further common analysis steps include filtering, normalization, identification of highly variable features, clustering, and identification of differentially expressed markers. Finally, the results are integrated with the spatial information to reveal patterns of differentiation in the different spatial features.
Spatial biology: challenges and the future
With multiple technologies still in the early development phase, a key practical challenge for spatial biology is how to increase the reproducibility and accuracy of results. Minor variations in sample handling, probe preparation, staining protocols, and instrumentation can introduce unwanted differences in results, particularly regarding coverage, background noise, and sensitivity.
Standardizing protocols and performing comparative analyses is complicated due to the wide range of possible parameters for protocol optimization (e.g. sample fixation/preservation, permeabilization, probe sequences, mass spectrometry matrix choice, ion polarity mode) and the unique alterations that may be necessary to obtain optimal results for specific tissues or custom instrument setups.
Key broader challenges also include:
- improving spatial resolution down to subcellular details
- increasing molecular coverage, throughput, and multiplexing
- developing standardized, integrated experimental and computational methods to jointly analyze multi-modal spatial data
- establishing comprehensive atlases spanning whole organisms
In addition, reliable and compact automation systems are needed to facilitate the screening of sample preparation conditions with custom protocols, while supporting different sample formats and delivering high reproducibility.
In conclusion, spatial omics is still in its infancy, so most methods and protocols are relatively new and expected to be substantially improved as they gain wider adoption.
Increasing access to automation, streamlining of advanced multi-step workflows, and the growing popularity of microfluidics, barcoding, and imaging mass spectrometry are improving scalability and reproducibility, driving the field forward.
By overcoming remaining technical barriers in throughput, resolution and integrated computational analysis, spatial omics promises to revolutionize our understanding of the spatial basis of cellular organization, developmental patterning and disease pathogenesis within intact tissues and organisms. Check out our next article to find out how to overcome some of these key challenges and make spatial omics a reality.
References
1. Alexandrov, T., Saez-Rodriguez, J., & Saka, S. K. (2023). Enablers and challenges of spatial omics, a melting pot of technologies. Molecular systems biology, 19(11), e10571.
Pubmed: https://pubmed.ncbi.nlm.nih.gov/37842805/
DOI: https://doi.org/10.15252/msb.202110571
2. Bressan, D., Battistoni, G., & Hannon, G. J. (2023). The dawn of spatial omics. Science (New York, N.Y.), 381(6657), eabq4964.
Pubmed: https://pubmed.ncbi.nlm.nih.gov/37535749/
DOI: https://doi.org/10.1126/science.abq4964
3. https://pubmed.ncbi.nlm.nih.gov/?term=%22spatial+omics%22&sort=date (Accessed 21 May 2024)
4. Method of the Year 2020: spatially resolved transcriptomics. Nat Methods 18, 1 (2021).
DOI: https://doi.org/10.1038/s41592-020-01042-x
5. , Larsson, L., Frisén, J., & Lundeberg, J. (2021). Spatially resolved transcriptomics adds a new dimension to genomics. Nature methods, 18(1), 15–18.
Pubmed : https://pubmed.ncbi.nlm.nih.gov/33408402/
DOI : https://doi.org/10.1038/s41592-020-01038-7
6. Marx, V. Method of the Year: spatially resolved transcriptomics. Nat Methods 18, 9–14 (2021).
DOI : https://doi.org/10.1038/s41592-020-01033-y
7. Zhuang X. (2021). Spatially resolved single-cell genomics and transcriptomics by imaging. Nature methods, 18(1), 18–22.
Pubmed : https://pubmed.ncbi.nlm.nih.gov/33408406/
DOI : https://doi.org/10.1038/s41592-020-01037-8
8. Rao, A., Barkley, D., França, G. S., & Yanai, I. (2021). Exploring tissue architecture using spatial transcriptomics. Nature, 596(7871), 211–220.
Pubmed : https://pubmed.ncbi.nlm.nih.gov/34381231/
DOI : https://doi.org/10.1038/s41586-021-03634-9
9. Moffitt, J. R., Lundberg, E., & Heyn, H. (2022). The emerging landscape of spatial profiling technologies. Nature reviews. Genetics, 23(12), 741–759.
Pubmed: https://pubmed.ncbi.nlm.nih.gov/35859028/
DOI: https://doi.org/10.1038/s41576-022-00515-3
10. Moses, L., & Pachter, L. (2022). Museum of spatial transcriptomics. Nature methods, 19(5), 534–546.
Pubmed: https://pubmed.ncbi.nlm.nih.gov/35273392/
DOI: https://doi.org/10.1038/s41592-022-01409-2
11. Palla, G., Spitzer, H., Klein, M. et al. Squidpy: a scalable framework for spatial omics analysis. Nat Methods 19, 171–178 (2022).
DOI: https://doi.org/10.1038/s41592-021-01358-2
12. Vandereyken, K., Sifrim, A., Thienpont, B., & Voet, T. (2023). Methods and applications for single-cell and spatial multi-omics. Nature reviews. Genetics, 24(8), 494–515.
Pubmed : https://pubmed.ncbi.nlm.nih.gov/36864178/
DOI : https://doi.org/10.1038/s41576-023-00580-2
13. Datta, S., Malhotra, L., Dickerson, R., Chaffee, S., Sen, C. K., & Roy, S. (2015). Laser capture microdissection: Big data from small samples. Histology and histopathology, 30(11), 1255–1269.
DOI: https://doi.org/10.14670/HH-11-622
14. Guo, W., Hu, Y., Qian, J., Zhu, L., Cheng, J., Liao, J., & Fan, X. (2023). Laser capture microdissection for biomedical research: towards high-throughput, multi-omics, and single-cell resolution. Journal of genetics and genomics = Yi chuan xue bao, 50(9), 641–651.
Pubmed: https://pubmed.ncbi.nlm.nih.gov/37544594/
DOI : https://doi.org/10.1016/j.jgg.2023.07.011
15. Eng, C. L., Lawson, M., Zhu, Q., Dries, R., Koulena, N., Takei, Y., Yun, J., Cronin, C., Karp, C., Yuan, G. C., & Cai, L. (2019). Transcriptome-scale super-resolved imaging in tissues by RNA seqFISH. Nature, 568(7751), 235–239.
Pubmed: https://pubmed.ncbi.nlm.nih.gov/30911168/
DOI: https://doi.org/10.1038/s41586-019-1049-y
16. Lu, T., Ang, C. E., & Zhuang, X. (2022). Spatially resolved epigenomic profiling of single cells in complex tissues. Cell, 185(23), 4448–4464.e17.
Pubmed : https://pubmed.ncbi.nlm.nih.gov/36272405/
DOI : https://doi.org/10.1016/j.cell.2022.09.035
17. Gyllborg, D., Langseth, C. M., Qian, X., Choi, E., Salas, S. M., Hilscher, M. M., Lein, E. S., & Nilsson, M. (2020). Hybridization-based in situ sequencing (HybISS) for spatially resolved transcriptomics in human and mouse brain tissue. Nucleic acids research, 48(19), e112.
Pubmed : https://pubmed.ncbi.nlm.nih.gov/32990747/
DOI : https://doi.org/10.1093/nar/gkaa792
18. Lee, H., Marco Salas, S., Gyllborg, D., & Nilsson, M. (2022). Direct RNA targeted in situ sequencing for transcriptomic profiling in tissue. Scientific reports, 12(1), 7976. Pubmed: https://pubmed.ncbi.nlm.nih.gov/35562352/
DOI: https://doi.org/10.1038/s41598-022-11534-9
19. Hu, K. H., Eichorst, J. P., McGinnis, C. S., Patterson, D. M., Chow, E. D., Kersten, K., Jameson, S. C., Gartner, Z. J., Rao, A. A., & Krummel, M. F. (2020). ZipSeq: barcoding for real-time mapping of single cell transcriptomes. Nature methods, 17(8), 833–843.
Pubmed: https://pubmed.ncbi.nlm.nih.gov/32632238/
DOI: https://doi.org/10.1038/s41592-020-0880-2
20. Kishi, J. Y., Liu, N., West, E. R., Sheng, K., Jordanides, J. J., Serrata, M., Cepko, C. L., Saka, S. K., & Yin, P. (2022). Light-Seq: light-directed in situ barcoding of biomolecules in fixed cells and tissues for spatially indexed sequencing. Nature methods, 19(11), 1393–1402.
Pubmed: https://pubmed.ncbi.nlm.nih.gov/36216958/
DOI: https://doi.org/10.1038/s41592-022-01604-1
21. Ali, H. R., Jackson, H. W., Zanotelli, V. R. T., Danenberg, E., Fischer, J. R., Bardwell, H., Provenzano, E., CRUK IMAXT Grand Challenge Team, Rueda, O. M., Chin, S. F., Aparicio, S., Caldas, C., & Bodenmiller, B. (2020). Imaging mass cytometry and multiplatform genomics define the phenogenomic landscape of breast cancer. Nature cancer, 1(2), 163–175.
Pubmed: https://pubmed.ncbi.nlm.nih.gov/35122013/
DOI: https://doi.org/10.1038/s43018-020-0026-6
22. Lin, J. R., Izar, B., Wang, S., Yapp, C., Mei, S., Shah, P. M., Santagata, S., & Sorger, P. K. (2018). Highly multiplexed immunofluorescence imaging of human tissues and tumors using t-CyCIF and conventional optical microscopes. eLife, 7, e31657.
Pubmed: https://pubmed.ncbi.nlm.nih.gov/29993362/
DOI: https://doi.org/10.7554/eLife.31657
23. Fang, S., Chen, B., Zhang, Y., Sun, H., Liu, L., Liu, S., Li, Y., & Xu, X. (2023). Computational Approaches and Challenges in Spatial Transcriptomics. Genomics, proteomics & bioinformatics, 21(1), 24–47.
Pubmed: https://pubmed.ncbi.nlm.nih.gov/36252814/
DOI: https://doi.org/10.1016/j.gpb.2022.10.001
Keywords:
About the author
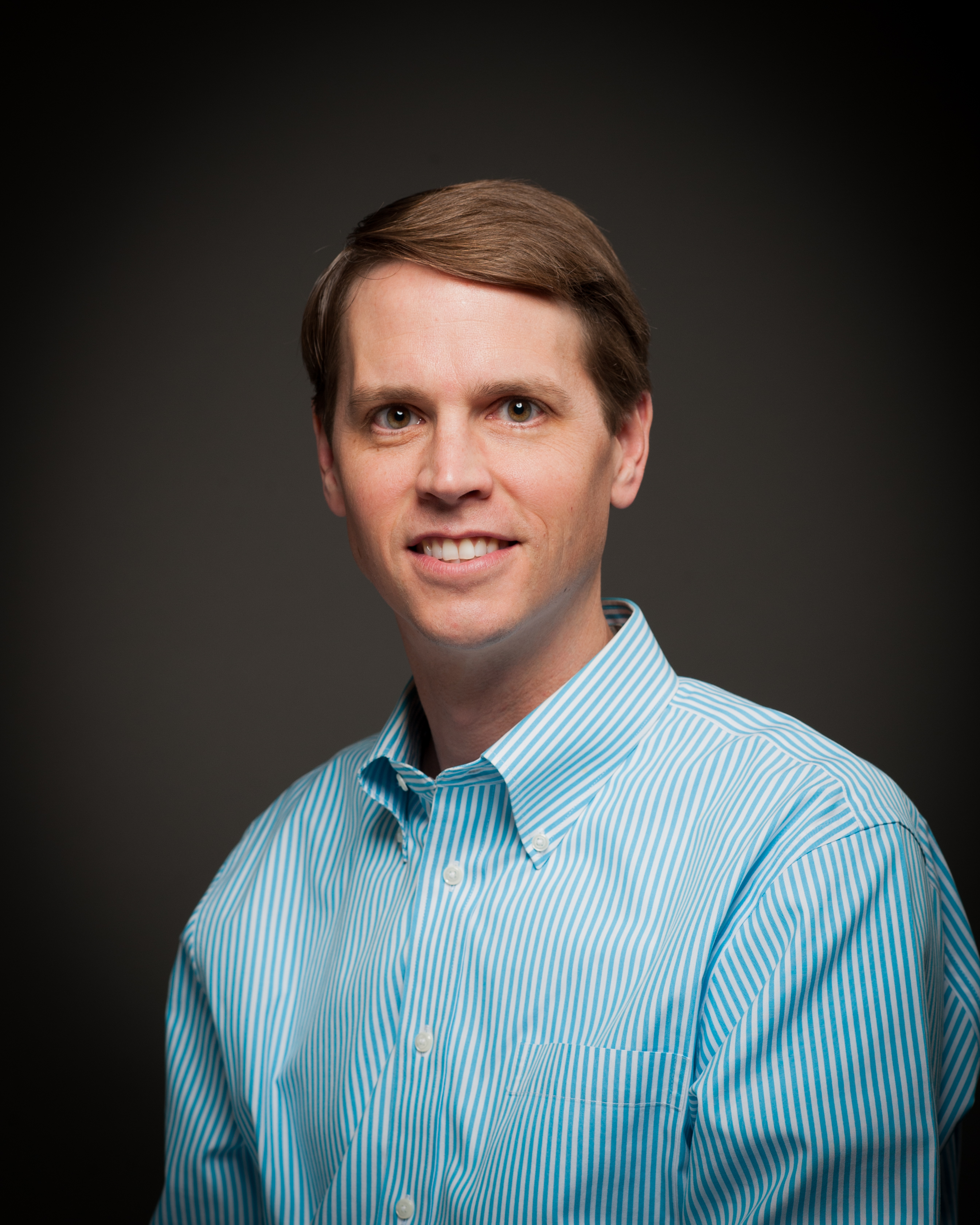
David Wold
David Wold is the Customer Operations Manager for the Partnering Business in the Components Marketing Team. In this role, he oversees a team responsible for the support and satisfaction of Tecan’s top Components customers. Since 2005, David has helped Tecan customers meet their liquid handling challenges across a broad range of technologies and applications. He brings understanding and expertise to help customer choose components to meet their needs, provide maximum reliability and outstanding performance.