By Magali Fischer
With more than 50% of preclinical results estimated to be irreproducible, the reliability of methods, assays, and protocols is a major concern in all areas of research. Many critical assay workflows, such as those for ELISA tests, are prone to error, even when using a high quality kit. While ELISA kits provide a solid basis to generate reliable data, troubleshooting the complete assay workflow is the first step toward pinpointing additional sources of variability and error that must be addressed in order to increase reproducibility and confident decision making.
Is poor data reproducibility compromising the reliability of your ELISA assays?
The burden of poor data reproducibility
Data reproducibility is crucial in regulated work such as pharma R&D and manufacturing, as well as clinical and academic research applications. Data rigor and reproducibility in clinical research is a systemic problem and a priority issue for many organizations, including the National Institutes of Health (NIH).¹ Errors in preclinical research can be very costly. The cumulative prevalence of irreproducible preclinical research has been estimated to exceed 50%, resulting in approximately US$28,000,000,000 (US$28B)/year spent on preclinical research that is not reproducible – in the United States alone.²
The data reproducibility problem is not limited to clinical research and the pharma industry. A report published in 2016 on reproducibility across many scientific fields shows that more than 70% of the over 1500 researchers contacted have tried and failed to reproduce another scientist's experiments, and more than half have failed to reproduce their own experiments.³ Such insights have led to a drive to implement quality control procedures in academic research.⁴
A case in point: the reproducibility of ELISA
High performance ELISA has become an invaluable method in clinical diagnostics and research and isregarded as a simple and low-cost way to measure analytes in a sample. Running ELISA has, however, traditionally been a time-consuming and laborious manual process that is relatively inefficient and error-prone. The need to quickly generate reliable data consistently, day-to-day is challenged by problems with variability within assays, between labs, and with low throughput.
Problems with manual ELISA can include:
- High background
- Weak or no signal, or too much signal
- Incorrect standard curves
- Poor replicates
- Inconsistency between assays
Causes can include:
- Poorly optimized assay
- Out-of-date reagents
- Not following dilution recommendations in kit instructions
- Incorrect pipetting technique
- Cross contamination
- Incorrect incubation temperatures
Correct manual pipetting
As an example of the complexity of manual tasks, we can consider some of the details needed to ensure correct manual pipetting:
1. Use the correct pipette and tip2. Ensure tip is firmly seated on the pipette
3. Ensure that there are no air bubbles while pipetting
4. Change tips between standards, samples, or reagents
5. Use dedicated reservoirs for each reagent
6. Pipette onto the side of wells to avoid splashing
These requirements are stringent, time consuming and may lead to operator fatigue. Furthermore, multiple rounds of accurate pipetting and thorough aspiration are required during the process.
Achieving reproducible results
There are also a number of points to remember in general to achieve reproducible assay results:
- Do not mix components from different kit lots
- Use a pipetting scheme to verify an appropriate plate layout
- Pipette in duplicate to be able to identify potential pipetting errors
- Do not modify test procedure or sample handling
- Do not exceed incubation times, temperatures or shaking
- Do not interrupt assay steps
- Handle all wells in the same order and time sequences.
- Avoid contamination of reagents, pipettes and wells/tubes
- Ensure washing steps are correctly performed
Subscribe to stay updated
In the next article in this series we will look at a case study that demonstrates how automation can address many of these issues, and help you improve the reproducibility of assays for biomarkers used to diagnose Alzheimer’s disease.
References
1. Prinz F, Schlange T, Asadullah K. Believe it or not: how much can we rely on published data on potential drug targets? Nat Rev Drug Discov. 2011; 10(9):712.
2. Freedman, L.P., Cockburn, I.M., and Simcoe, T.S. (2015) The Economics of Reproducibility in Preclinical Research. PLoS Biol. 13(6): e1002165.
3. Baker, M. (2016) 1500 scientists lift the lid on reproducibility. Nature 533: 452–454.
4. Baker, M. (2016) How quality control could save your science. Nature 529: 456–458.
About the author
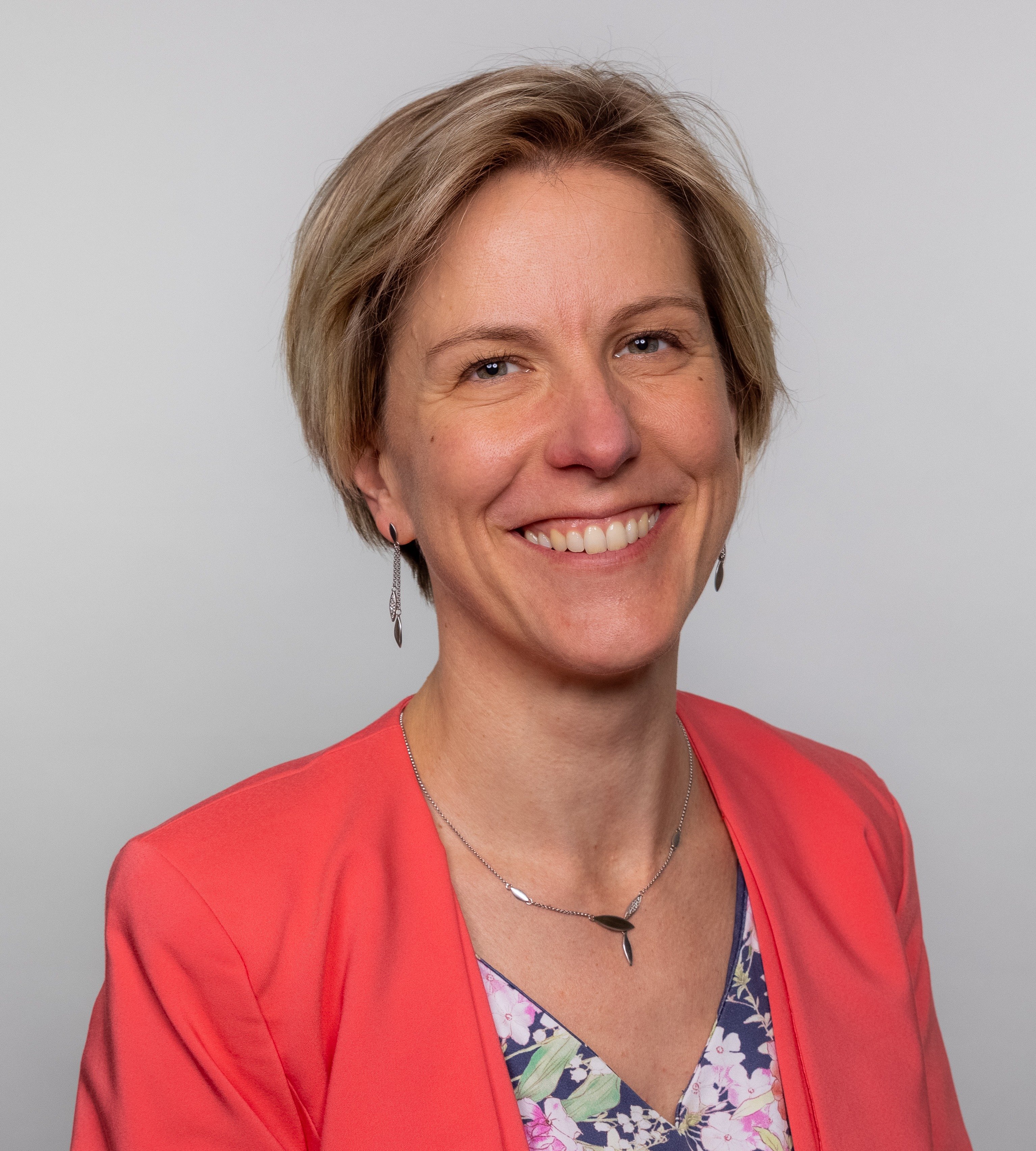
Magali Wolff
Magali Wolff is the Head of Global Reagent Marketing and Support at Tecan. She has been with the company since 2001. Magali and her team of product managers are dedicated to providing Tecan’s customers with the best service and quality products for their daily workflows.