By Kevin Moore
Data driven decision-making depends on generating reliable data in a timely fashion. But the reproducibility of biomedical research results, or rather lack of it, has become a big issue. A recent Nature survey¹ revealed a “reproducibility crisis” in the research community, with 70% of respondents having failed to reproduce the work of other researchers, and over half even failing to reproduce their own results.
Could your research be resting on shaky foundations?
With many underlying causes, this crisis will not be resolved overnight. However, significant progress can be made by adopting tools and strategies that minimize human error, while improving standardization and record keeping. Automation can address many of these challenges, improving the reproducibility and trustworthiness of your data, and at the same time increasing the efficiency of your research.
Reproducible data inspires confidence
Publishing erroneous results can have serious and far-reaching consequences, not only for the originating laboratory but also for the journal that accepts the faulty work and for the many scientists who may cite the work and use it as the basis for their own experimental research. Lack of repeatability and reproducibility in preclinical research can cause significant delays in the development of lifesaving therapies and may be costing up to $28 billion in the US alone².
Solutions to the reproducibility problem are many, including adoption of best practices in laboratory work, sound experimental design, protocol standardization, the use of high quality reagents and reference materials, and implementation of automation to minimize error. Indeed, automation has many advantages beyond those normally associated with routine lab work, such as increasing throughput and minimizing hands-on time.
Automation is therefore moving into the academic world, as pharma and biotech are outsourcing more drug discovery and translational research to academic drug discovery labs. Automation is enabling the development of robust and reliable workflows that meet the requirements of generating reproducible data from new and complex translational research methods.
Automation minimizes costly sample and data loss
Generating reproducible data is important, but losing data can be catastrophic. Automation brings with it the security of knowing that your samples will go into the correct wells and reagents will be dispensed accurately. The result is tight data with fewer outliers, and automation may well reduce the number of repeat assays you need to run. For complex studies with many samples, automated barcoding can prevent mix-ups, and ensure reliable data tracking and documentation. In addition, automation facilitates larger scale studies with more statistical power to detect subtle effects reliably.
Success in lead generation also relies on dependable and pain-free automated compound management. Methods such as next generation sequencing rely on a highly dependable automated workhorse that can support genomic workflows with reproducible hands-off sample prep.
How to be fast AND reliable
Complexity of experimental methods and pressure to produce results quickly are common explanations for data that cannot be reproduced. As a researcher in drug discovery you may well be asked to get a better angle of attack on complex questions by adopting and adapting a wide range of methods. Analytical methodologies are diversifying all the time to accommodate emerging technologies such as CRISPR/CAS9 analysis, live cell-based assays, next generation sequencing, and high throughput screening.
Drug discovery workflows also require technologies that can support diverse current and future applications. These may involve multiple microplate formats, liquid transfers of different volume ranges, varying detection read-outs including fluorescence and luminescence, and even label-free techniques such as mass spectrometry.
With this diversity in mind, automation could well help you gain control over your workload and enable you to generate high quality, trustworthy data in a timely manner. But the world of research is highly dynamic, bringing inevitable changes during projects. While accuracy and reproducibility are vital regardless of the application, working to consistently high standards should not mean being locked into the inertia of lab workflows and protocols that are difficult to modify. Ease of use and flexibility are key.
In the next post in this series we will look in more detail into how researchers are using automation to increase their production of reliable and reproducible data.
References
1. 1,500 scientists lift the lid on reproducibility. Baker M. Nature. 2016 May 26;533(7604):452-4. doi: 10.1038/533452a. https://www.nature.com/news/1-500-scientists-lift-the-lid-on-reproducibility-1.19970 | Pubmed >
2. The Economics of Reproducibility in Preclinical Research. Freedman LP, Cockburn IM, and Simcoe TS. PLoS Biol. 2015 Jun 9;13(6):e1002165. doi: 10.1371/journal.pbio.1002165. eCollection 2015 Jun. http://journals.plos.org/plosbiology/article?id=10.1371/journal.pbio.1002165#sec010 | Pubmed >
Want to stay updated?
Subscribe to the Tecan blog to receive the latest articles and ideas straight to your inbox.
About the author
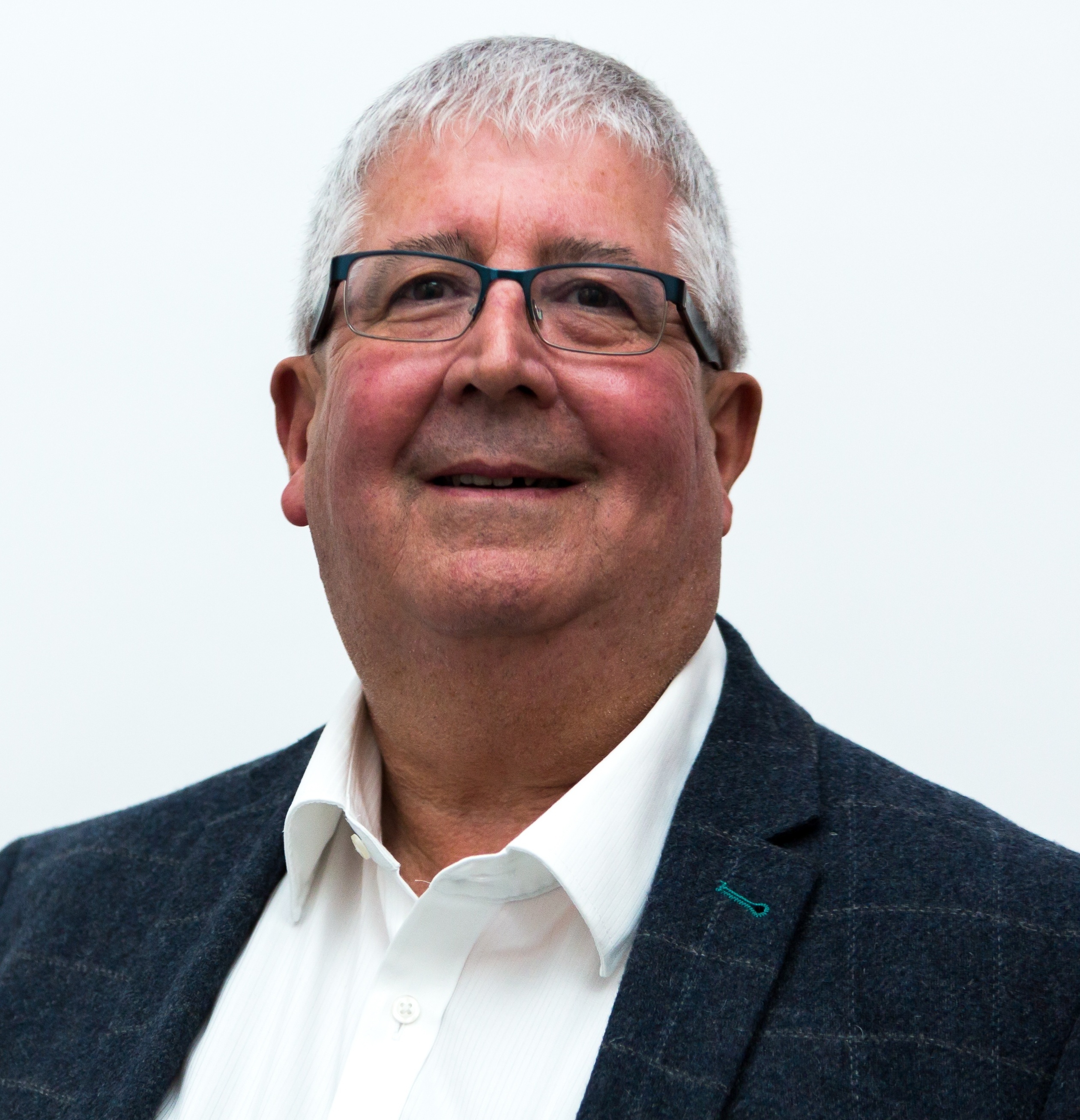
Kevin Moore
Kevin Moore is Head of Markets and Applications based out of Tecan’s head office in Männedorf, Switzerland. He heads the team tasked with bringing both products and application for the liquid handling to the market. Prior to joining Tecan in 2007, he was head of Compound Management and Technology project manager for the Neuroscience Research Centre of Merck & Co in the UK, where he worked for Merck for 20 years.