By Nicholas Smith
Cognitive computing and artificial intelligence have the power to save us from drowning in the vast and growing sea of data needed for precision medicine, but what will it take to achieve a timely return on investment? Experts from multiple disciplines will gather to share their perspectives on this challenging problem at the upcoming Tecan Symposium in Salt Lake City on November 14th.
Can artificial intelligence rescue precision medicine?
The future of precision medicine and digital health should be bright. Recent technological advances are helping scientists unravel the molecular mechanisms of human health and disease at a remarkable rate. Staggering amounts of data are being amassed to profile individuals based on genes, lifestyle and environment. But there’s a dark side: the data is growing to proportions that defy human analysis, and much of it may be useless in its current form.
Data, data everywhere
Precision medicine is fueled by ‘big data’. To determine the right course of treatment for the right person at the right time, detailed patient profiles must be compiled from diverse information. The results then need to be stratified and correlated with clinical outcomes, cross-checking with pharmaceutical and biomedical databases. The success of precision medicine will depend on how quickly clinicians can identify patterns in the data and make relational inferences.
The emergence of cost-effective, high-resolution sequencing technologies has led to an explosion of genetic data. Base and sequence data registered with GenBank has increased exponentially over the past 35 years, doubling approximately every 18 months¹. The number of WGS bases in GenBank now exceeds two trillion, with at least one projection estimating that it will increase by up to five orders of magnitude over the coming decade.
In addition to genetic sequences, useful data can come from a variety of different sources. These can be as diverse as physicians’ notes, patient surveys, medical images, in vitro diagnostic test results and wearable sensors.
Battling the dark side
Unfortunately, as much as 80% of relevant healthcare information may be so-called ‘dark’ data that cannot be accessed for analysis². To be useful, the data needs to be tagged and structured so that it can be processed appropriately. Data coming from disparate sources needs to be harmonized so that it can be combined and correlated. Importantly, all data must be rigorously generated and quality checked to avoid errors that could have devastating consequences for patients.
An industry brief from Dell EMC and IDC identified healthcare as “one of the fastest growing segments of the digital universe”³. With an annual growth rate of 48%, even if the data is 100% structured, harmonized, and error-free, the size and complexity of the database will soon defy human analysis.
Raising the IQ of precision medicine
Fortunately, the acceleration of precision medicine intersects with the dawning of an exciting era in cognitive computing and artificial intelligence (AI). Over the past five years especially, AI supercomputers like Watson have impressed us with their ability to out-think human experts, making intelligent and complex decisions without human assistance.
Significant breakthroughs in cognitive computing have been made by turning from traditional rule-based approaches to deep learning. Advances in pattern recognition, language processing and data mining strategies are revolutionizing the field.
Today’s AI supercomputers can make quadrillions of calculations per second. These petascale speeds (and more) are what will be needed to tame the big data that underpins precision medicine.
With billions of dollars committed to ambitious healthcare initiatives, the stakes are high for precision medicine. Cognitive computing holds great promise for faster and more reliable decision-making, but good solutions cannot be developed in isolation. Clinicians, researchers, physicians, bioinformatics specialists and other experts must come together to drive the right outcomes.
References
- NCBI website: GenBank and WCS Statistics, Accessed 29 Oct 2017. https://www.ncbi.nlm.nih.gov/genbank/statistics/
- “Unstructured data in electronic health record (EHR) systems: challenges and solutions.” Whitepaper, published 10 Jan 2017. Accessed 29 Oct 2017. https://insights.datamark.net/white-papers/unstructured-data-in-electronic-health-record-systems-challenges-and-solutions
- “The digital universe driving data growth in healthcare.” Vertical industry brief with research and analysis by IDC. Accessed 29 Oct 2017. https://www.emc.com/analyst-report/digital-universe-healthcare-vertical-report-ar.pdf
About the author
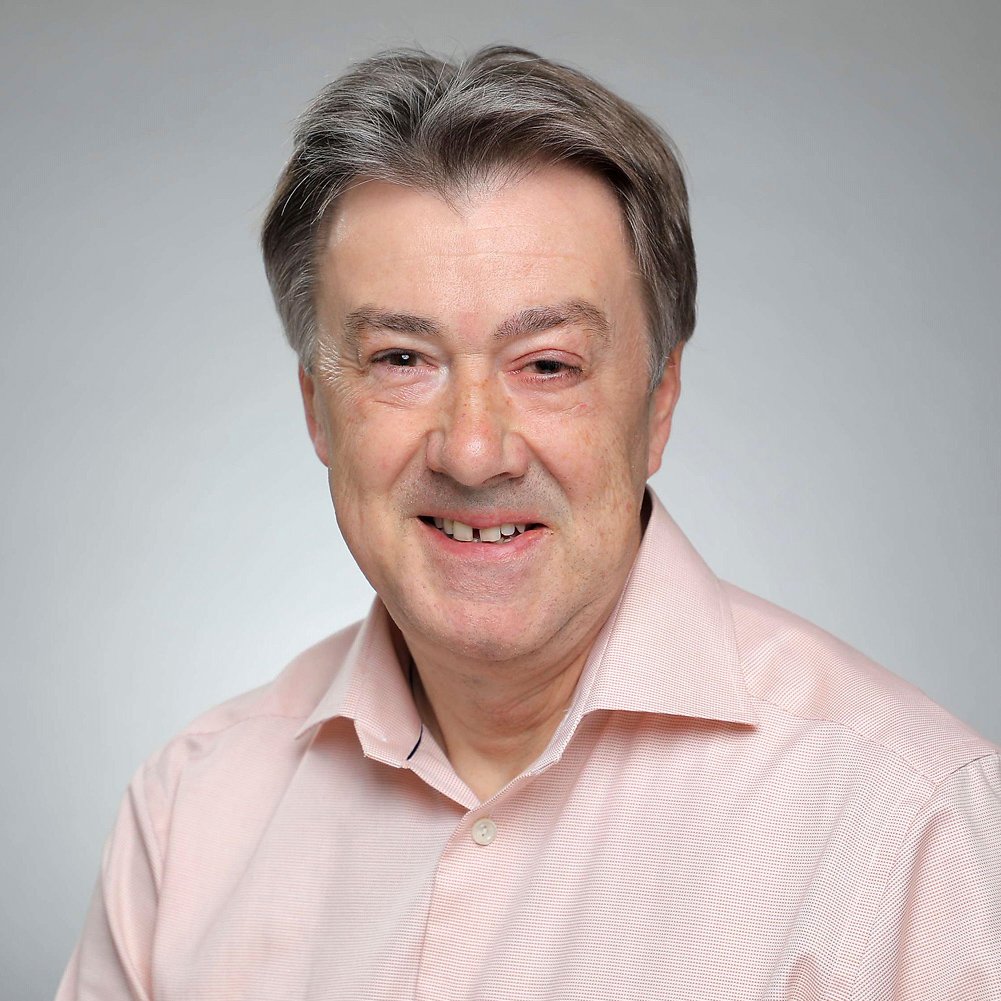
Nicholas Smith
Nicholas Smith is Head of Global Marketing and Portfolio Management for Tecan’s Partnering Business. A key function of his team is to work closely with customers to develop new product concepts and proposals based on a thorough analysis of their specific requirements. Nicholas’ joined Tecan in 2012 from Roche Diagnostics where he worked for over 20 years in a variety of roles within marketing and business development.